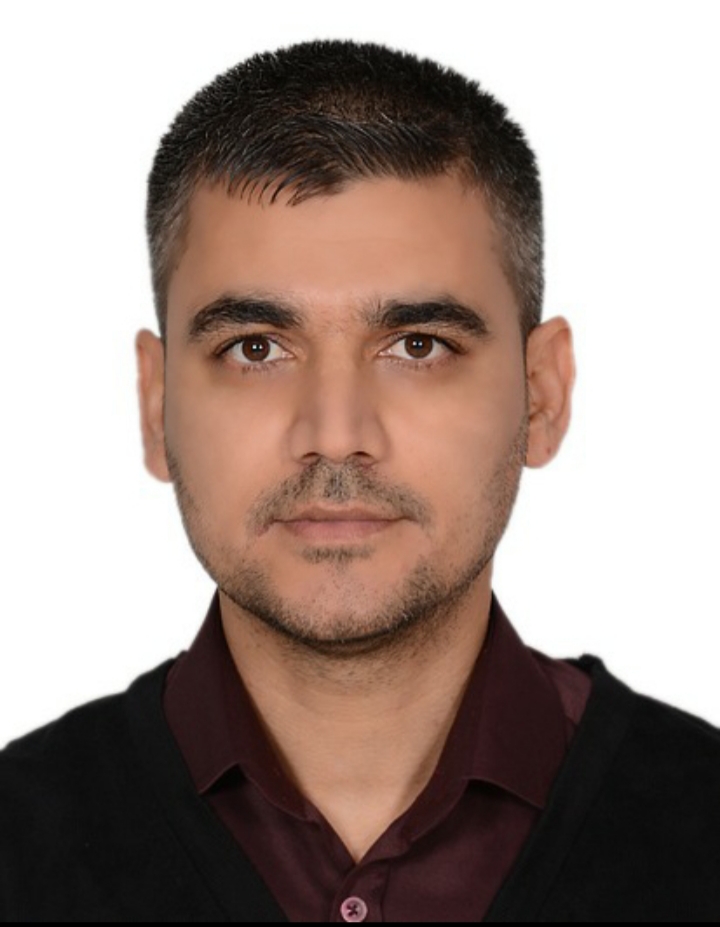
Imad Burhan Kadhim
Research InterestsElectronics
Control
Communications and Electronic Circuits
Gender | MALE |
---|---|
Place of Work | Kirkuk Technical Institute |
Position | . |
Qualification | Master |
Speciality | Electrical and computer engineering/Electronics and control |
emad.burhan86@ntu.edu.iq | |
Phone | 07717988581 |
Address | kirkuk, kirkuk, kirkuk, Iraq |
Skills
استخدام الحاسوب (75%)
استخدام برامج الوورد والاكسل (75%)
مهارة التدريس بطرق مختلفة (90%)
Working Experience
. [مسؤول وحدة التاهيل والتوظيف والمتابعة]
Nov 5, 2020 - Presentايجاد فرص عمل للطلبة الخريجين واقامة دورات وندوات وورش في جميع الاختصاصات لكسب المهارة في مجال عملهم
. [مسؤول شعبة شؤون الطلبة]
Oct 13, 2022 - Presentقبول الطلبة في الدراستين الصباحية والمسائية وتزويد الطلبة الخريجين بالتاييدات والوثائق
Publications
Reinforcement Learning for Speech Recognition using Recurrent Neural Networks
Aug 26, 2022Journal 2022 2nd Asian Conference on Innovation in Technology (ASIANCON) Pune, India. Aug 26-28, 2022
publisher 1st Imad Burhan Kadhim 2nd Mahdi Fadil Khaleel 3rd Zuhair Shakor Mahmood 4th Ali Najdet Nasret Coran
Volume conference
This work describes a voice recognition system that does not need an intermediate phonetic representation to convert audio input to text. The system is based on a mix of the the Connectionist Temporal Classification goal function and deep bidirectional LSTM recurrent neural network architecture . A new method is proposed in which the network is taught to reduce the likelihood of an arbitrary transcription loss function being encountered. without the aid of any lexicons or models, this allows for a direct optimization of WER. The system has a WER (word error rate) of 22 percent , 20 percent with simply a lexicon of authorized terms, 9 percent using a trigram language model. The error rate drops to 7 percent when the network is used in conjunction with a baseline system. Index Terms—word error rate, deep bidirectional, recurrent neural network
Enhancement and modification of automatic speaker verification by utilizing hidden Markov model
Oct 15, 2022Journal Indonesian Journal of Electrical Engineering and Computer Science
publisher Imad Burhan Kadhim, Ali Najdet Nasret, Zuhair Shakor Mahmood
DOI 10.11591/ijeecs.v27.i3.pp1397-1403
Volume 27
The purpose of this study is to discuss the design and implementation of autonomous surface vehicle (ASV) systems. There’s a lot riding on the advancement and improvement of ASV applications, especially given the benefits they provide over other biometric approaches. Modern speaker recognition systems rely on statistical models like hidden Markov model (HMM), support vector machine (SVM), artificial neural networks (ANN), generalized method of moments (GMM), and combined models to identify speakers. Using a French dataset, this study investigates the effectiveness of prompted text speaker verification. At a context-free, single mixed mono phony level, this study has been constructing a continuous speech system based on HMM. After that, suitable voice data is used to build the client and world models. In order to verify speakers, the text-dependent speaker ver- ification system uses sentence HMM that have been concatenated for the key text. Normalized log-likelihood is determined from client model forced by Viterbi algorithm and world model, in the verification step as the difference between the log- likelihood. At long last, a method for figuring out the verification results is revealed.