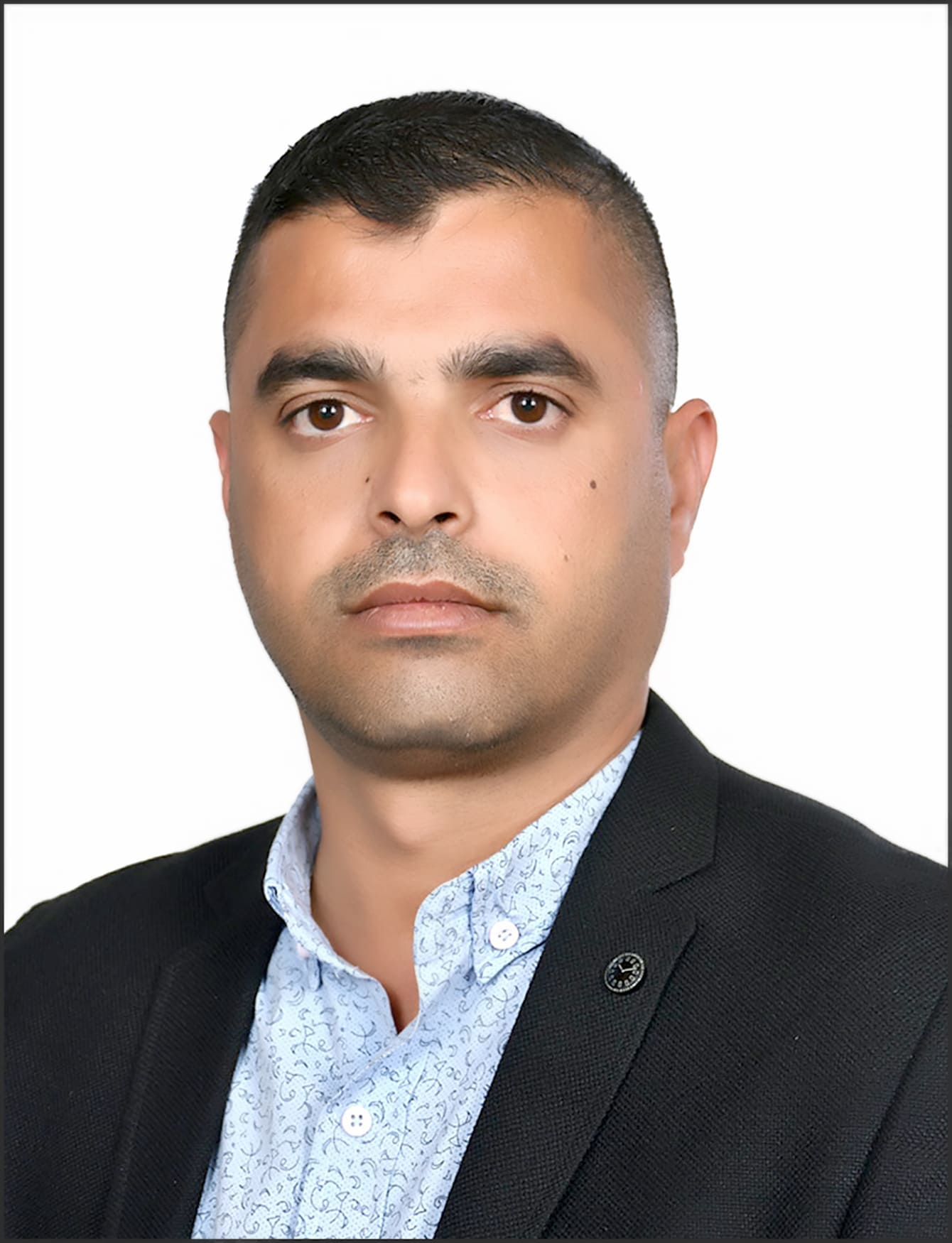
Mohanad Mohammed Rashid
Research InterestsDeep Learning
Machine Learning
Data Mining
Gender | MALE |
---|---|
Place of Work | Mosul Medical Technical Institute |
Position | Department coordinator |
Qualification | Master |
Speciality | Computer science |
mohanad.rashid@ntu.edu.iq | |
Phone | 07736847318 |
Address | Mosul, Mosul, Mosul, Iraq |
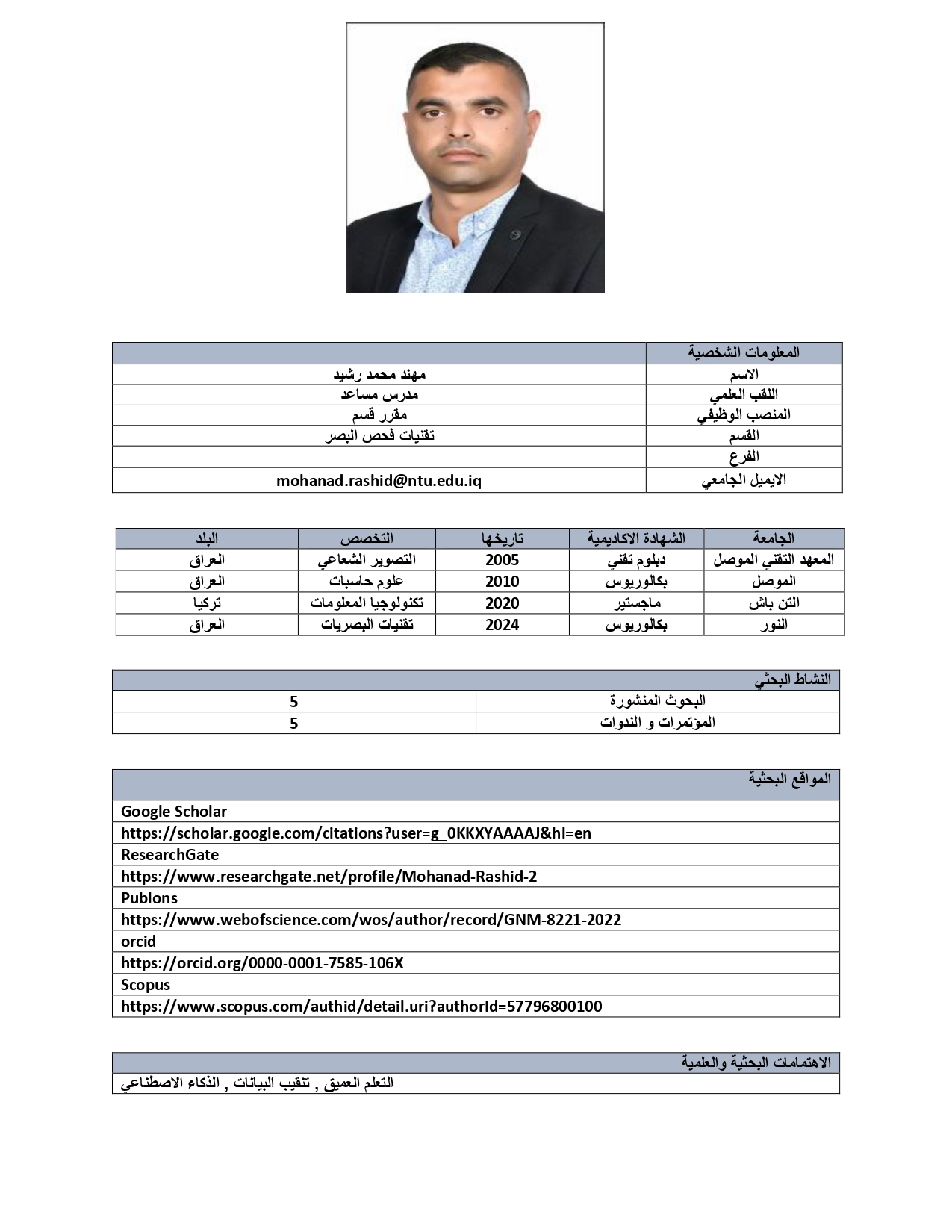
Academic Qualification
Master
Jan 20, 2019 - Jan 26, 2022Information Technology
B.S.c
Oct 1, 2006 - Oct 1, 2010Computer Science
Technical Diploma
Oct 1, 2003 - Oct 1, 2005Radiography
B.S.c
Oct 1, 2020 - Jun 30, 2024Optical Techniques
Publications
An Improved Ensemble Machine Learning Approach for Diabetes Diagnosis
Apr 24, 2024Journal PERTANIKA JOURNAL OF SCIENCE & TECHNOLOGY
publisher Universiti Putra Malaysia Press
DOI https://doi.org/10.47836/pjst.32.3.19
Issue 3
Volume 32
Diabetes is recognized as one of the most detrimental diseases worldwide, characterized by elevated levels of blood glucose stemming from either insulin deficiency or decreased insulin efficacy. Early diagnosis of diabetes enables patients to initiate treatment promptly, thereby minimizing or eliminating the risk of severe complications. Although years of research in computational diagnosis have demonstrated that machine learning offers a robust methodology for predicting diabetes, existing models leave considerable room for improvement in terms of accuracy. This paper proposes an improved ensemble machine learning approach using multiple classifiers for diabetes diagnosis based on the Pima Indians Diabetes Dataset (PIDD). The proposed ensemble voting classifier amalgamates five machine learning algorithms: Decision Tree (DT), Logistic Regression (LR), K-Nearest Neighbor (KNN), Random Forests (RF), and XGBoost. We obtained the individual model accuracies and used the ensemble method to improve accuracy. The proposed approach uses a pre-processing stage of standardization and imputation and applies the Local Outlier Factor (LOF) to remove data anomalies. The model was evaluated using sensitivity, specificity, and accuracy criteria. With a reported accuracy of 81%, the proposed approach shows promise compared to prior classification techniques.
Detection of Retinal Detachment Using Deep Learning and Data Mining Approaches
Jan 7, 2025Journal International Journal of Multidisciplinary Research and Growth Evaluation
DOI https://doi.org/10.54660/.IJMRGE.2025.6.1-1743-1748
Issue 1
Volume 6
Retinal detachment (RD) is a critical ocular condition requiring prompt diagnosis to prevent permanent vision loss. Delays in detection can lead to irreversible damage, highlighting the need for accurate and efficient diagnostic methods. This study presents a novel deep-learning framework integrated with advanced image processing techniques for RD diagnosis, utilizing optical coherence tomography (OCT) scans as the primary imaging modality. The proposed hybrid convolutional neural network (CNN) architecture combines feature fusion from multiple layers with adaptive image enhancement tailored to RD-specific characteristics. Key contributions include implementing wavelet-based noise reduction, adaptive histogram equalization, and edge-aware segmentation to preprocess OCT images effectively. An ensemble-based CNN model was also designed to extract multi-scale features and prioritize RD-relevant regions through attention mechanisms. The model achieved a classification accuracy of 97.8%, sensitivity of 97.4%, specificity of 98.6%, and an AUC-ROC of 98.7%, surpassing benchmarks in retinal diagnostics. The study also compares the proposed framework with state-of-the-art methods, demonstrating its superior performance in robustness and interpretability. These findings pave the way for deploying advanced diagnostic tools in clinical practice, enhancing early detection and treatment of retinal detachment.
An Explainable Artificial Intelligence (XAI) Methodology for Heart Disease Classification
Jan 10, 2025Journal International Journal of Current Science Research and Review
DOI https://doi.org/10.54660/.IJMRGE.2025.6.1-1743-1748
Issue 2
Volume 8
Heart disease continues to be one of the predominant contributors to morbidity and mortality on a global scale, underscoring the imperative for early and precise diagnosis to enhance patient outcomes. Machine Learning (ML) has emerged as a formidable instrument in the classification of cardiovascular diseases, utilizing intricate clinical datasets to discern patterns that conventional statistical methodologies may fail to detect. Nevertheless, notwithstanding their robust predictive capabilities, numerous machine learning models function as black-box systems, exhibiting a deficiency in transparency regarding their decision-making processes. The absence of interpretability presents a considerable challenge in clinical environments, where trust, accountability, and elucidation are of utmost importance for medical professionals. In order to tackle this issue, we propose a methodology for heart disease classification that is grounded in Explainable Artificial Intelligence (XAI). This approach incorporates interpretable machine learning models to improve diagnostic transparency and reliability. Our framework conducts an evaluation of various classifiers, including Support Vector Machine (SVM), Gradient Boosting (GB), Extreme Gradient Boosting (XGBoost), Multi-Layer Perceptron (MLP), and LightGBM. This assessment is based on essential performance metrics, namely accuracy, precision, recall, F1-score, and AUC-ROC. Furthermore, SHAP (SHapley Additive exPlanations) and LIME (Local Interpretable Model-Agnostic Explanations) have been integrated to enhance the interpretability of the model. The experimental findings indicate that XGBoost surpasses alternative models, attaining the highest classification accuracy of 92% and an AUC-ROC score of 0.93, all while preserving interpretability. This study underscores the significance of incorporating Explainable Artificial Intelligence (XAI) techniques within medical AI applications. It advocates for the adoption of transparent, interpretable, and clinically dependable machine learning methodologies to enhance clinical decision-making and optimize patient outcomes.
AI-Driven Cybersecurity Measures for Hybrid Cloud Environments: A Framework for Multi-Cloud Security Management
Jan 30, 2025Journal International Journal on Engineering Artificial Intelligence Management, Decision Support, and Policies
Issue 1
Volume 2
The complex landscape of multi-cloud settings is the result of the fast growth of cloud computing and the ever-changing needs of contemporary organizations. Strong cyber defenses are of fundamental importance in this setting. In this study, we investigate the use of AI in hybrid cloud settings for the purpose of multi-cloud security management. For hybrid cloud deployment, this mathematical approach maximizes a security metric. Programmers use backslashes to escape special characters and identify file paths. The objective function optimizes application and data asset security by using AI-enhanced security solutions across all cloud providers, taking their weights into consideration. The conclusion is that hybrid cloud environments can benefit greatly from AI-enabled multi-cloud security management. Using a combination of mathematical modeling and data-driven AI techniques, we were able to dynamically adjust our security allocations in real-time response to evolving threats, optimize effective resourcing for threat incident response based on continuous risk assessment/prediction, learning from past incidents as well as feedforward modeling. Although the paper studies hypothetical data, it provides a nicely conceptual basis for using empirical data in the real world and this study is an important step towards navigating practical implications in cloud security management a sentence.
Conferences
APPLICATIONS OF MACHINE LEARNING IN BATTLING AGAINST NOVEL COVID-19
Jun 9, 2022 - Jun 11, 2022Publisher IEEE
DOI https://doi.org/10.1109/HORA55278.2022.9799969
Country Turkey
Location Ankara