 معاملات.jpeg)
Israa Tahseen Ali Al-Attar
Research InterestsInternet of Things
Web programming
Artificial Intelligence
Smart Cities
Network Security
Network Protocols
Multimedia
Gender | FEMALE |
---|---|
Place of Work | Technical Engineering College/ Kirkuk |
Position | Assist. Prof. |
Qualification | Ph.d |
Speciality | Computer Networks and Internet Programming |
israa.ali24@ntu.edu.iq | |
Phone | +964 (770) 272 3461 |
Address | Erbil, Iraq, Erbil, Erbil, Iraq |
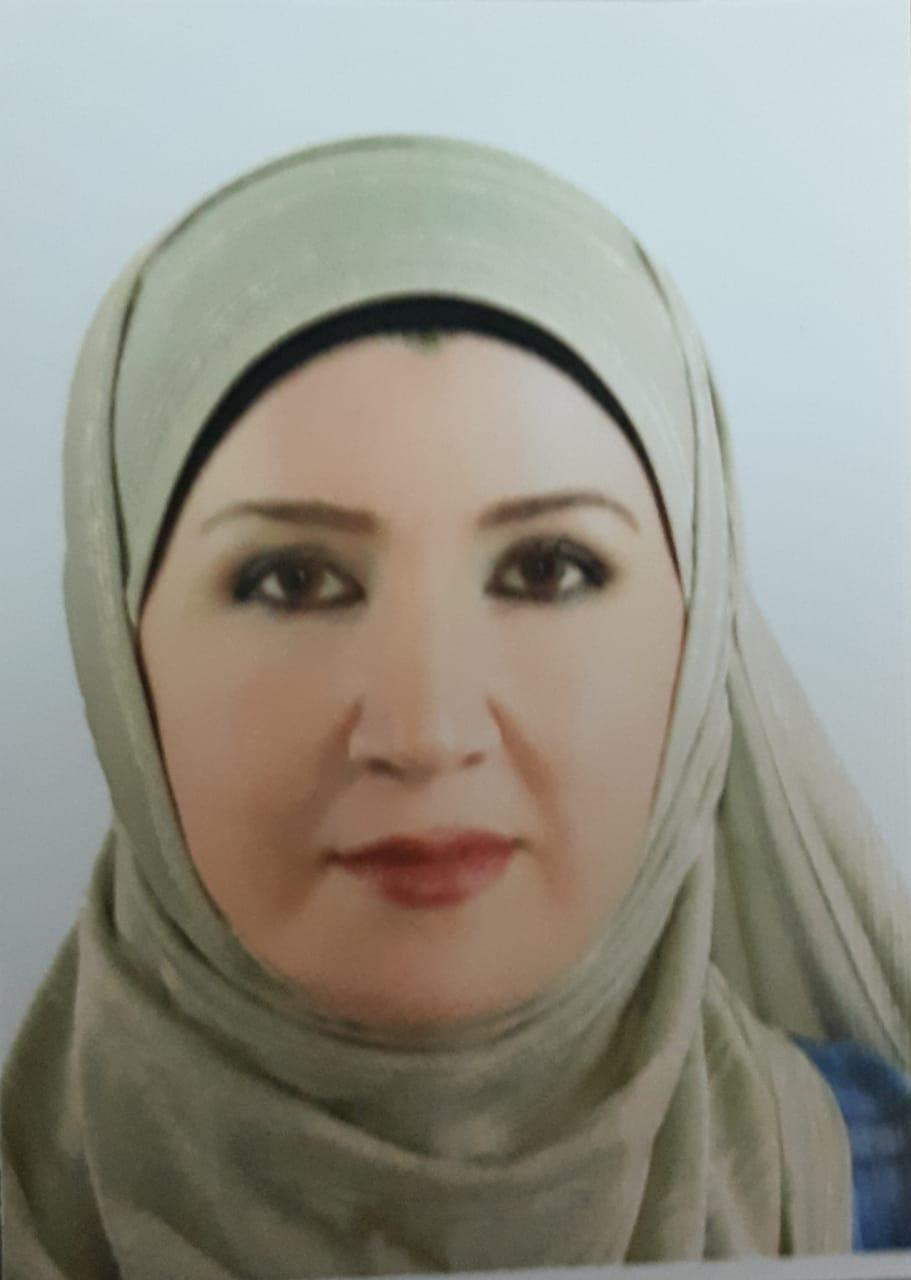
Israa Tahseen Ali is an Iraqi academic specialized in networks and artificial intelligence. She was born in Baghdad in 1980. She earned her Bachelor's degree in Computer Science from University of technology in 2002, followed by a Master's degree in the same field in 2005. In 2009, she completed her PhD in Computer Science at the same university.
Israa currently works as a University Professor at the Northern Technical University, where she teaches courses in networks and artificial intelligence. She also supervises Master's and PhD students, as well as undergraduate thesis projects.
Her research has been published in several local and international journals, and she has participated in various conferences focused on networks and artificial intelligence. She is particularly interested in developing artificial intelligence solutions to improve network systems and manage smart cities.
Israa is fluent in Arabic, Turkish, and English, with basic conversational skills in French. Her future goal is to integrate sustainability into networks and artificial intelligence fields to promote smart and sustainable solutions.
Skills
Internet applications programming (90%)
Supervision of applied projects (98%)
Preparing and Instructing scientific lectures in the field of computer technology (100%)
Design and development of curricula related to Internet and network application programming (100%)
Supervision
masoud
Year: 2010Academic Degree: Bachelor
Supervisor Type: Supervisor
Supervisor State: Graduated
Batool
Year: 2014Academic Degree: Master
Supervisor Type: Supervisor
Supervisor State: Graduated
Image Search Engine Design
Duaa Salim
Year: 2015Academic Degree: Master
Supervisor Type: Supervisor
Supervisor State: Graduated
Deep Search Engin
Shaymaa Jawad
Year: 2015Academic Degree: Master
Supervisor Type: Supervisor
Supervisor State: Graduated
Image Processing , Steganography, Hole Filling Techniques
Adnan Tahir
Year: 2015Academic Degree: Master
Supervisor Type: Co-supervisor
Supervisor State: Graduated
Olaa Amer
Year: 2015Academic Degree: Master
Supervisor Type: Co-supervisor
Supervisor State: Graduated
Zahraa Jawad
Year: 2016Academic Degree: Master
Supervisor Type: Co-supervisor
Supervisor State: Graduated
Noor Aldeen Mohammed
Year: 2017Academic Degree: Master
Supervisor Type: Supervisor
Supervisor State: Graduated
Khitam
Year: 2018Academic Degree: Master
Supervisor Type: Co-supervisor
Supervisor State: Graduated
safa mushab
Year: 2019Academic Degree: Master
Supervisor Type: Supervisor
Supervisor State: Graduated
Taif Rafee
Year: 2019Academic Degree: Master
Supervisor Type: Co-supervisor
Supervisor State: Graduated
Mohammed
Year: 2020Academic Degree: PhD
Supervisor Type: Co-supervisor
Supervisor State: Graduated
Mohammed Radthi
Year: 2021Academic Degree: Master
Supervisor Type: Supervisor
Supervisor State: Graduated
Hussein Kutaiba
Year: 2022Academic Degree: PhD
Supervisor Type: Co-supervisor
Supervisor State: In Progress
Sarah Hussein
Year: 2023Academic Degree: PhD
Supervisor Type: Co-supervisor
Supervisor State: In Progress
dania, najim,
Year: 2024Academic Degree: Bachelor
Supervisor Type: Supervisor
Supervisor State: In Progress
Academic Qualification
Dectorate
Sep 1, 2005 - Jul 1, 2009PhD in Computer Science / University of Technology
Master
Sep 1, 2002 - May 1, 2005Msc. in Computer Science / University of Technology
Bachelors
Sep 1, 1998 - Jun 25, 2002Bachelors in Computer Science / University of Technology
Working Experience
researcher at Department Computer Science / University of Technology [Researcher]
Oct 5, 2002 - May 1, 2005Follow up on students' implementation of programs while developing their programming skills in scientific laboratories.
Asst. Lecturer at Department of Computer Science / University of Technology [Asst. Lecturer]
May 1, 2005 - Dec 31, 2007Preparing and Instructing lectures on programming languages and several fundamentally courses.
Asst. Lecturer at Department of software engineering / University of Saladdin, Erbil, Iraq [Asst. Lecturer]
Jan 1, 2008 - Dec 15, 2008Preparing and Instructing lectures on programming languages and several fundamentally courses.
Asst. Lecturer at Department of Computer Science / University of Technology [Asst. Lecturer / rapporteur of Artificial Intelligence Department]
Dec 16, 2008 - Sep 30, 2009Lecturer at Department of Computer Science / University of Technology [Lecturer / rapporteur of Artificial Intelligence Department]
Oct 1, 2009 - Aug 15, 2011Lecturer at Department of Computer Science / University of Technology [Lecturer / Head of Computer Networks Department]
Aug 16, 2011 - Oct 12, 2013Lecturer at Department of Computer Science / University of Technology [Lecturer]
Oct 22, 2013 - Jul 31, 2017Asst. Prof at Department of Computer Science / University of Technology [Asst Prof. / Head of Artificial Intelligence Department]
Aug 1, 2017 - Jul 1, 2020Asst. Prof. at Department of Computer Science / University of Technology [Asst. Prof.]
Jul 2, 2020 - Jul 31, 2024Asst. Prof. at Department of Computer Techniques engineering / Northern Technical University, Kirkuk, Iraq [Asst. Prof.]
Aug 1, 2024 - PresentPublications
Survey of Multiple Destination Route Discovery Protocols
Jul 16, 2024Journal International Journal of Computational and Experimental Science and Engineering
publisher Prof.Dr. İskender AKKURT
Issue 3
Volume 10
Route discovery protocols for multiple destinations are one of the most interesting research topics since it is applied for real-world applications and is needed in smart city services such as delivery services. The inclusion of artificial intelligence can improve the performance of multiple destination route discovery protocols. In this paper, we studied and analyzed multiple destination route discovery protocols based on different search strategies, especially artificial intelligence methods. The survey compares multiple destination route discovery protocols related to their applications and implementation tools. Important parameters are considered regarding route planning such as the mapping models of multiple destinations and different artificial intelligence search strategies. In this survey route discovery protocols for multiple destinations consider their different goals related to travel time and cost deadlines, moving obstacles, real-time traffic conditions in the city, customer satisfaction, and optimal route. In conclusion, using artificial intelligence can enhance route discovery protocols for multiple destinations compared to traditional search methods.
An Enhancement for Wireless Body Area Network Using Adaptive Algorithms
Jul 16, 2024Journal International Journal of Computational and Experimental Science and Engineering
publisher Prof.Dr. İskender AKKURT
Issue 3
Volume 10
Wireless Body Area Networks (WBANs) are one of the most critical technologies for maintaining constant monitoring of patient’s health and diagnosing diseases. They consist of small, wearable wireless sensors transmitting signals. Within this vision, WBANs are not without unique difficulties, for instance, high energy consumption, heat from the sensor, and impaired data accuracy. This paper introduces adaptive algorithms combining Convolutional Neural Networks (CNNs) and dynamic threshold mechanisms to enhance the performance and energy efficiency of Wireless Body Area Networks. The study utilizes the MIB-BIH Arrhythmias dataset to improve the detection of arrhythmias. The results show a 10.53% improvement in battery life and a 5.62-fold enhancement in temperature management when sleep mode technology is applied. As a result, the model reached the average accuracy of ECG classification of 98% and a high level of selectivity and sensitivity to a normal type of heartbeat and quite satisfactory results in the classification of arrhythmia type of heartbeat
Traffic Accident Prediction Using Historical Accident Data and Machine Learning
Feb 19, 2025Traffic accidents continue to pose a significant public safety challenge, resulting in substantial human and economic losses around the globe. The primary goal of this study is to investigate the role of machine learning methodologies in predicting road traffic accidents through the examination of previous accident reports. The plan is to craft a predictive model by analyzing past accident occurrence trends and patterns that will flag potentially high-risk regions and periods for road traffic accidents. The data will come from various sources including police documents, traffic camera footage, and climate models, ensuring that it is not affected during this stage of the project. Different methods of machine learning like the decision tree model, random forests, and neural networks will be put through their paces in order to ascertain which among them gives the best prediction results. The outcomes show that the models we examined can be efficient traffic safety mechanisms linking decision-makers and the public as well as enabling timely targeted interventions. Finally, the research emphasizes the need to make good use of the historical data in developing advanced predictive systems, thus serving as an effective way of regulating road safety through forecasting.
Analyzing Credit Risk with Machine Learning for Financial Institutions
Feb 19, 2025Journal Conference
In recent years, the financial industry has increasingly adopted machine learning models to enhance traditional credit risk assessment methods. This paper explores the application of machine learning techniques in analyzing credit risk for financial institutions, emphasizing predictive accuracy and model interpretability. The study evaluates various algorithms, including logistic regression, decision trees, and ensemble methods such as random forests and boosting algorithms like gradient boosting, using a dataset of historical credit data. Key performance metrics are discussed to assess the effectiveness of these models in predicting default probabilities and managing risk. The paper also tackles the challenges posed by model complexity, regulatory compliance, and ethical considerations regarding the use of machine learning models. The research results indicate that while machine learning models are more effective in risk prediction, proper implementation and continuous checking are necessary to ensure fair credit decision-making while preventing model biases. Thus, this paper adds to the existing literature that supports the inclusion of advanced analytics in financial risk management practices.
Predicting Judicial Decisions Using Machine Learning on Historical Court Case Data
Feb 19, 2025Journal Conference
The judicial system plays a crucial role in the functioning of society, however, the process of predicting judicial decisions continues to be a difficult and critical task. This paper looks at how machine learning techniques can be applied to predict judicial outcomes based on historical court case data. The analysis utilizes datasets containing case summaries, legal arguments, and previous verdicts, which can be also outfitted with sophisticated analytical models to enable the identification of patterns and critical factors which most likely impacted verdicts. The approach comprehensively includes data preprocessing, feature extraction, and the leveraging of advanced machine learning methods like natural language processing for text analysis. The findings show that the models can achieve a maximum precision rate in predicting the outcomes of cases, thus, giving legal practitioners, policymakers, and researchers remarkable knowledge. In addition, this study includes the discussions of ethical issues, limitations, and how to improve the level of legal transparency and efficiency. The project aspires to contribute to the rising area of research on the integration of law and artificial intelligence which also includes the development of predictive analytics for justice and the judicial process with the employment of this research methodology of data mining.
Conferences
Predicting Judicial Decisions Using Machine Learning on Historical Court Case Data
Feb 18, 2025 - Feb 19, 2025Country Pakistan
Location Sindh Agriculture University, Tandojam
Analyzing Credit Risk with Machine Learning for Financial Institutions
Feb 18, 2025 - Feb 19, 2025Country Pakistan
Location Sindh Agriculture University, Tandojam
Traffic Accident Prediction Using Historical Accident Data and Machine Learning
Feb 18, 2025 - Feb 18, 2025Country Pakistan
Location Sindh Agriculture University, Tandojam