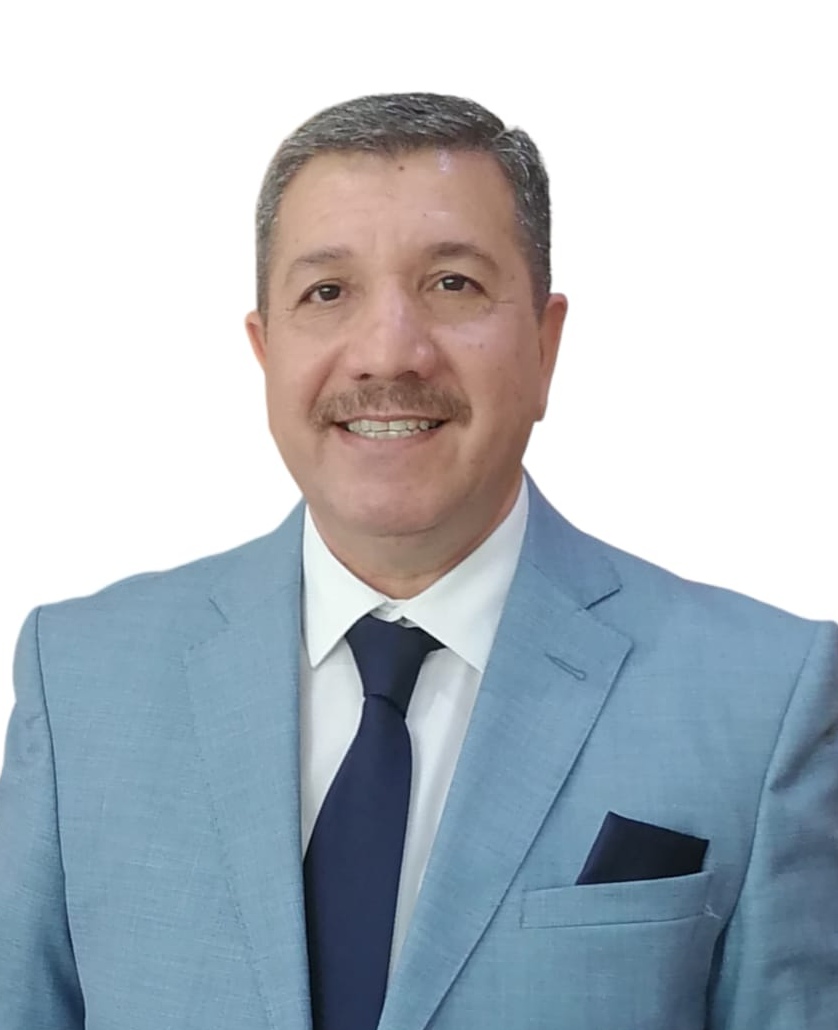
Hasan Kareem Abdulrahman
Research InterestsPattern recognition
Machine Learning
Color image steganalysis
Edge detection and Evaluation
Gender | MALE |
---|---|
Place of Work | Kirkuk Technical Institute |
Position | Head of Computer Systems Techniques Department |
Qualification | Ph.D |
Speciality | Image processing and information Security |
Hasan.abdulrahman@ntu.edu.iq | |
Phone | +9647730501500 |
Address | Iraq, Kirkuk, Iraq, Mosul, Iraq |
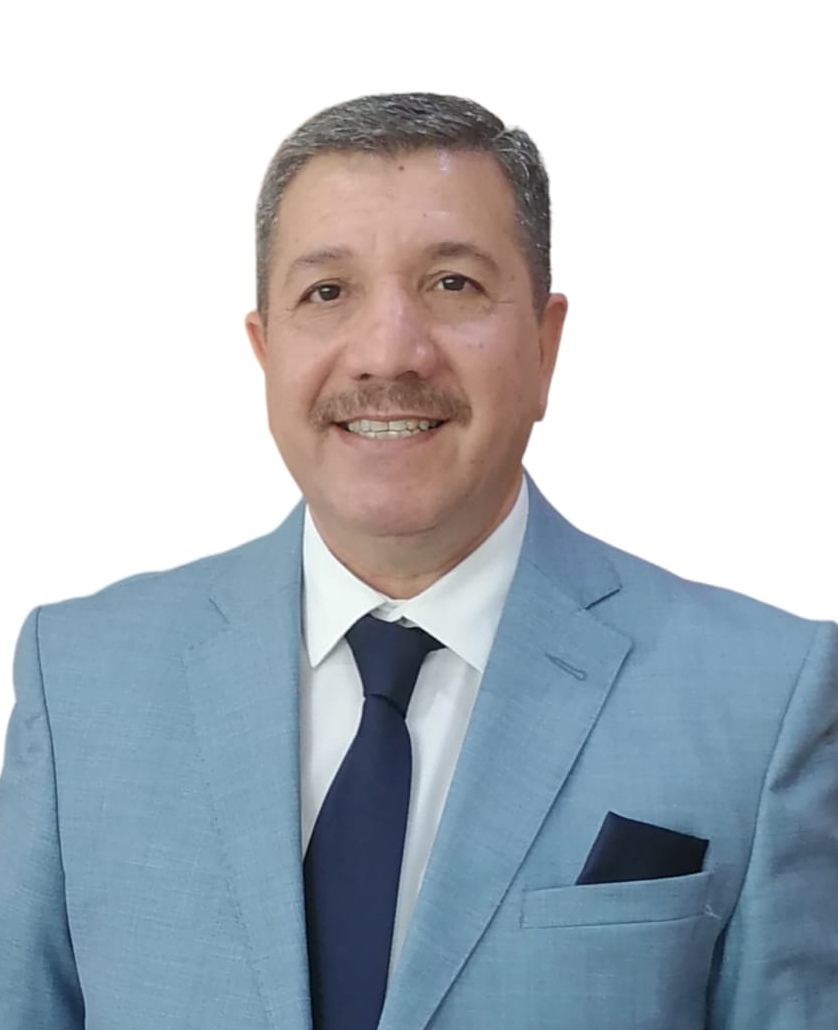
HASAN ABDULRAHMAN: He received the Ph.D. degree in computer science from University of Montpellier, France in 2017. He is currently working as an Associate Professor Head of Department of Computer techniques systems, at the Northern Technical University. He has received the Postdoctoral 2023-2024 France, Campus France/144091Y. He has authored or co-authored many research papers in international journals (SCI/Wiley/Scopus) and conferences, including IEEE, Elsevier and Springer conference proceeding. He has presented various papers at international conferences and his paper in WSCG2017 was selected as one of best paper awards.
He doing his research at the IMT Mines Ales, laboratory (CERIS) Centre denseignement et de recherche en informatique et systemes in France. In cooperation with University of Montpellier. His current research interests include information security, steganography, steganalysis, cyber security, computer vision, deep learning, artificial intelligence, image processing, big data, and Biometric systems.
Languages
English – TOEFL-612, (88%)
French – DELF-B2, (90%)
Kurdish mother language (100%)
Arabic (100%)
Skills
Oracle (80%)
PhP (80%)
Java (86%)
html (87%)
Kali Linux (90%)
Python (90%)
Vb (91%)
Foxpro (93%)
C++ (98%)
Academic Qualification
BSc.
Sep 1, 1988 - Jul 15, 1992BSc. of Science in Computer Science / Al-Mustansiriya University / Baghdad / Iraq
Master
Sep 1, 2013 - Dec 30, 2005Master of Science in Computer Science / University of Technology / Baghdad / Iraq
PhD.
Apr 13, 2014 - Nov 17, 2017PhD in Computer Science / University of Montpellier / France
Post-Doc.
Sep 1, 2023 - Jan 3, 2024Post-Doc. in Computer Science / University of Montpellier / France
Working Experience
Computer Systems [Head of Computer Systems Depatment/Asst.Prof. Dr.]
Sep 1, 2024 - PresentPost-Doc [Post-Doc/ University of Montpllier]
Sep 1, 2023 - Mar 1, 2024Asst.Prof.Dr. [Head of Computer Tech. Systems Depatment]
Sep 1, 2019 - Sep 1, 2022Assistant Dean [Assistant Dean for Student Affairs]
Sep 1, 2022 - Feb 27, 2023Assistant Dean [Assistant Dean for Administrative and Financial Affairs]
Sep 1, 2009 - Jan 10, 2013Publications
From Contours to Ground Truth: How to Evaluate Edge Detectors by Filtering
Jul 13, 2017Journal Journal of WSCG
publisher Journal of WSCG
Issue No.1-2
Volume Volume: 25,
Edge detection remains a crucial stage in numerous image processing applications. Thus, an edge detection tech- nique needs to be assessed before use it in a computer vision task. As dissimilarity evaluations depend strongly of a ground truth edge map, an inaccurate datum in terms of localization could advantage inaccurate precise edge detectors or/and favor inappropriate a dissimilarity evaluation measure. Hence, in this work, we demonstrate how to label these ground truth data in a semi-automatic way. Moreover, several referenced-based boundary detection evaluations are detailed and applied toward an objective assessment. Thus, each measure is compared by varying the threshold of the thin edges. Indeed, theoretically, the minimum score of the measure corresponds to the best edge map, compared to the ground truth. Finally, experiments on many images using six edge detectors show that the new ground truth database allows an objective comparison of numerous dissimilarity measures
From Contours to Ground Truth: How to Evaluate Edge Detectors by Filtering
Jul 13, 2017Journal Journal of WSCG
publisher Journal of WSCG
Issue No.1-2
Volume Volume: 25,
Edge detection remains a crucial stage in numerous image processing applications. Thus, an edge detection tech- nique needs to be assessed before use it in a computer vision task. As dissimilarity evaluations depend strongly of a ground truth edge map, an inaccurate datum in terms of localization could advantage inaccurate precise edge detectors or/and favor inappropriate a dissimilarity evaluation measure. Hence, in this work, we demonstrate how to label these ground truth data in a semi-automatic way. Moreover, several referenced-based boundary detection evaluations are detailed and applied toward an objective assessment. Thus, each measure is compared by varying the threshold of the thin edges. Indeed, theoretically, the minimum score of the measure corresponds to the best edge map, compared to the ground truth. Finally, experiments on many images using six edge detectors show that the new ground truth database allows an objective comparison of numerous dissimilarity measures
A review of supervised edge detection evaluation methods and an objective comparison of filtering gradient computations using hysteresis thresholds
May 31, 2018Journal Journal of Imaging
publisher MDPI
Issue 1
Volume 4
Useful for human visual perception, edge detection remains a crucial stage in numerous image processing applications. One of the most challenging goals in contour detection is to operate algorithms that can process visual information as humans require. To ensure that an edge detection technique is reliable, it needs to be rigorously assessed before being used in a computer vision tool. This assessment corresponds to a supervised evaluation process to quantify differences between a reference edge map and a candidate, computed by a performance measure/criterion. To achieve this task, a supervised evaluation computes a score between a ground truth edge map and a candidate image. This paper presents a survey of supervised edge detection evaluation methods. Considering a ground truth edge map, various methods have been developed to assess a desired contour. Several techniques are based on the number of false positive, false negative, true positive and/or true negative points. Other methods strongly penalize misplaced points when they are outside a window centered on a true or false point. In addition, many approaches compute the distance from the position where a contour point should be located. Most of these edge detection assessment methods will be detailed, highlighting their drawbacks using several examples. In this study, a new supervised edge map quality measure is proposed. The new measure provides an overall evaluation of the quality of a contour map by taking into account the number of false positives and false negatives, and the degrees of shifting. Numerous examples and experiments show the importance of penalizing false negative points differently than false positive pixels because some false points may not necessarily disturb the visibility of desired objects, whereas false negative points can significantly change the aspect of an object. Finally, an objective assessment is performed by varying the hysteresis thresholds on contours of real images obtained by filtering techniques. Theoretically, by varying the hysteresis thresholds of the thin edges obtained by filtering gradient computations, the minimum score of the measure corresponds to the best edge map, compared to the ground truth. Twenty-eight measures are compared using different edge detectors that are robust or not robust regarding noise. The scores of the different measures and different edge detectors are recorded and plotted as a function of the noise level in the original image. The plotted curve of a reliable edge detection measure must increase monotonously with the noise level and a reliable edge detector must be less penalized than a poor detector. In addition, the obtained edge map tied to the minimum score of a considered measure exposes the reliability of an edge detection evaluation measure if the edge map obtained is visually closer to the ground truth or not. Hence, experiments illustrate that the desired objects are not always completely visible using ill-suited evaluation measure.
Authentication System Based Palmprint Recognition Using Simple Structured Neural Network
Oct 1, 2022Journal Asian Journal of Convergence in Technology
publisher Asian Journal of Convergence in Technology
Issue 2350-1146 I.F-5.11
Volume Volume VIII and Issue II
Biometrics technology is gaining popularity every day. In most countries nowadays, academics are focusing their efforts on biometrics because it has an important function in security. Analyzing a large number of security cases provided researchers with significant motivation to conduct additional research and develop new ideas. Biometrics technology also has multiple uses outside of the security industry, including civil, commercial, and industrial applications. Biometrics measures and analyzes unique physical and behavioral characteristics of people. Palmprints are currently considered the preferred biometric for application in highly sensitive access control environments such as federal buildings, airports, and other critical locations. Palmprint contains a more distinctive feature and does not require a high-resolution palmprint image, unlike other biometric characteristics. This paper involves designing of high accuracy palm recognition system using simple structured neural network called single hidden layer neural network. accuracy score for the proposed state of the art is found 91.3 %.
Conferences
A New Normalized Method of Object Shape-based Recognition and Localization
Jul 8, 2019 - Jul 10, 2019Publisher IET Computer Vision Journal
Country France
Location Tours, France
ntegrated Convolutional Neural Network Model With Statistical Moments Layer for Vehicle Classification
Nov 16, 2019 - Nov 18, 2019Publisher Published in SPIE Book
Country Netherland
Location Amsterdam